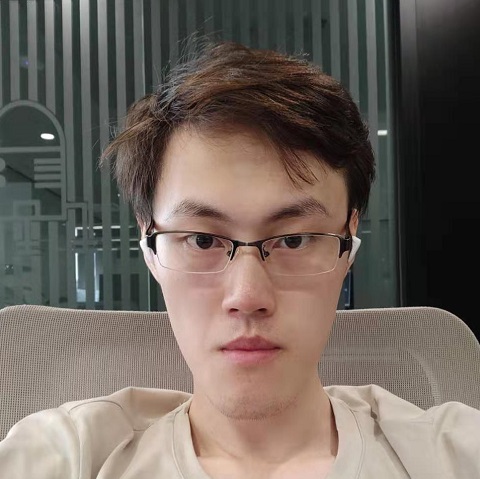
Reseacher Interests
- 2D/3D Face Recognition
- 2D/3D Face Anti-Spoofing
- 3D Face Reconstruction
Publications
Led3D: A Lightweight and Efficient Deep Approach to Recognizing Low-quality 3D Faces
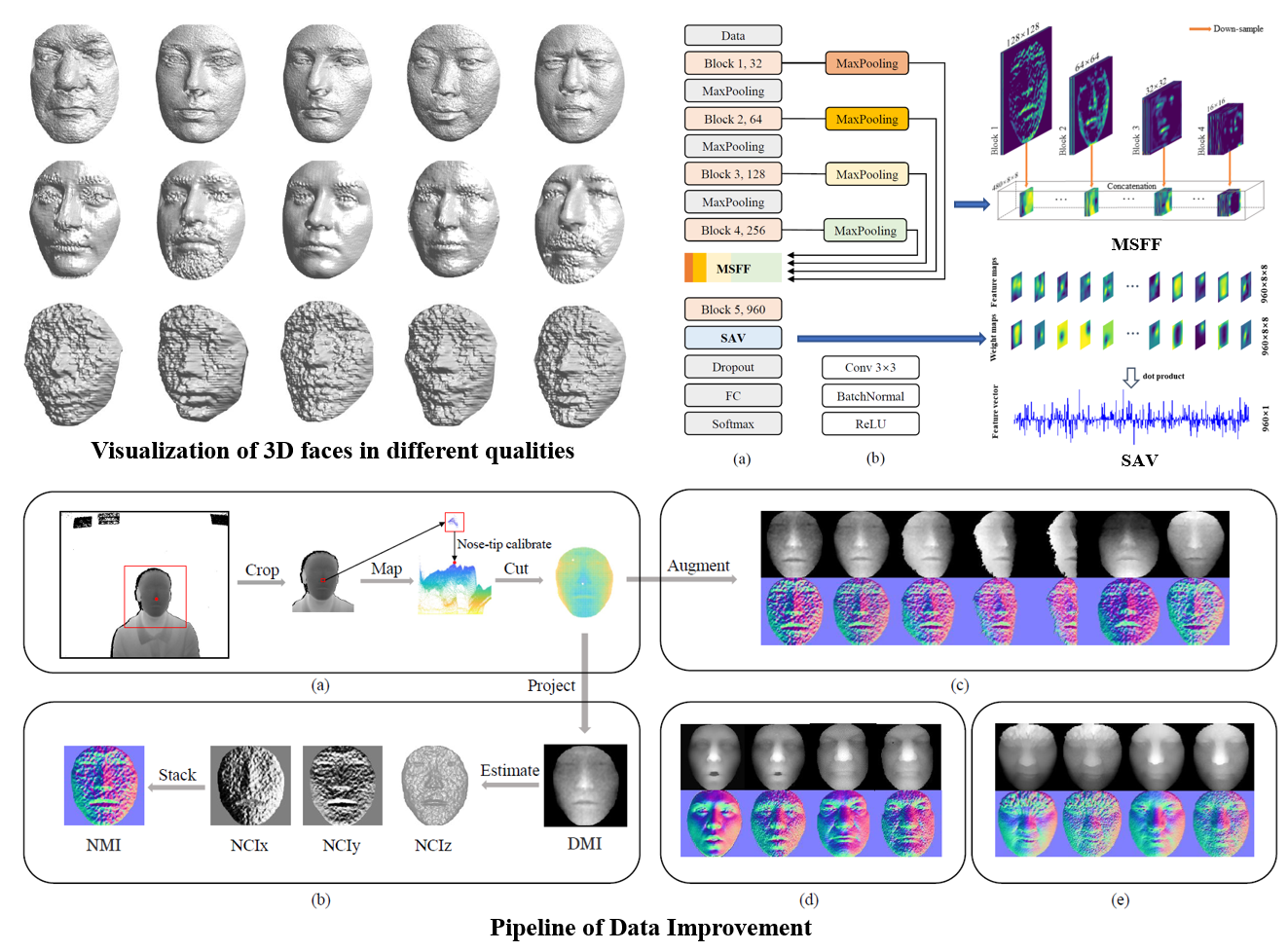
Due to the intrinsic invariance to pose and illumination changes, 3D Face Recognition (FR) has a promising potential in the real world. 3D FR using high-quality faces, which are of high resolutions and with smooth surfaces, have been widely studied. However, research on that with low-quality input is limited, although it involves more applications. In this paper, we focus on 3D FR using lowquality data, targeting an efficient and accurate deep learning solution. To achieve this, we work on two aspects: (1) designing a lightweight yet powerful CNN; (2) generating finer and bigger training data. For (1), we propose a Multi-Scale Feature Fusion (MSFF) module and a Spatial Attention Vectorization (SAV) module to build a compact and discriminative CNN. For (2), we propose a data processing system including point-cloud recovery, surface refinement, and data augmentation (with newly proposed shape jittering and shape scaling). We conduct extensive experiments on Lock3DFace and achieve state-of-the-art results, outperforming many heavy CNNs such as VGG-16 and ResNet-34. In addition, our model can operate at a very high speed(136 fps) on Jetson TX2, and the promising accuracy and efficiency reached show its great applicability on edge/mobile devices.
Keywords:
- 3DFR
- Jetson TX2
Facial Expression Synthesis by U-Net Conditional Generative Adversarial Networks
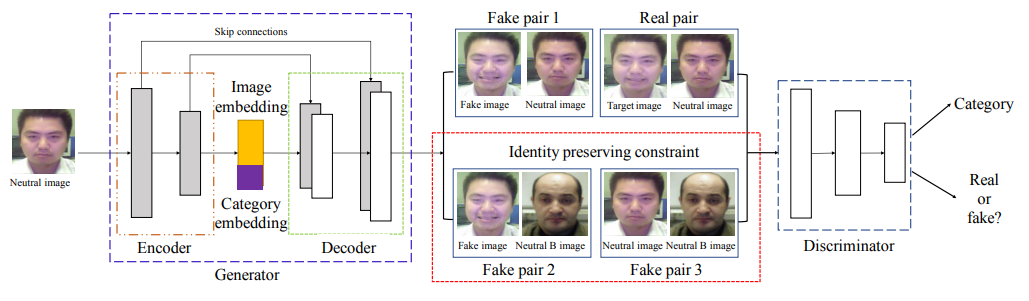
High-level manipulation of facial expressions in images such as expression synthesis is challenging because facial expression changes are highly non-linear, and vary depending on the facial appearance. Identity of the person should also be well preserved in the synthesized face. In this paper, we propose a novel U-Net Conditioned Generative Adversarial Network (UC-GAN) for facial expression generation. U-Net helps retain the property of the input face, including the identity information and facial details. We also propose an identity preserving loss, which further improves the performance of our model. Both qualitative and quantitative experiments are conducted on the Oulu-CASIA and KDEF datasets, and the results show that our method can generate faces with natural and realistic expressions while preserve the identity information. Comparison with the state-of-the-art approaches also demonstrates the competency of our method.
Keywords:
- U-Net
- Facial Expression
Education
-
MSc in Computer Science and TechnologyBeihang University2017 - 2020
-
BSc in Intelligent Science and TechnologyDalian Martime University2013 - 2017